Data Analysis and Reporting
Data analysis and reporting are critical components of the decision-making process within organizations. They involve the examination and interpretation of data to extract meaningful insights and inform business strategies. Here are key aspects of data analysis and reporting:
Data Analysis:
Data Collection:
Gathering relevant data from various sources, including databases, spreadsheets, sensors, and external APIs.
Data Cleaning and Preprocessing:
Cleaning and organizing raw data to address inconsistencies, missing values, and other issues that could affect analysis.
Exploratory Data Analysis (EDA):
Preliminary analysis to understand the characteristics of the data, identify patterns, and generate hypotheses.
Descriptive Statistics:
Calculating and summarizing basic statistics such as mean, median, mode, and standard deviation to describe the central tendency and variability of the data.
Data Visualization:
Creating visual representations of data through charts, graphs, and dashboards to facilitate easier understanding and interpretation.
Statistical Analysis:
Applying statistical methods to identify relationships, correlations, and patterns within the data.
Predictive Modeling:
Building models to make predictions or forecasts based on historical data, using techniques such as regression analysis and machine learning.
Hypothesis Testing:
Evaluating hypotheses and making statistical inferences to validate or reject assumptions about the data.
Text and Sentiment Analysis:
Analyzing textual data to extract insights, sentiments, and trends using natural language processing (NLP) techniques.
Spatial Analysis:
Examining geographic or spatial data to identify patterns or relationships based on location.
Data Reporting:
Dashboard Creation:
Developing dashboards that provide a visual overview of key performance indicators (KPIs) and metrics.
Report Design:
Creating clear and concise reports that communicate findings and insights effectively to various stakeholders.
Automation:
Automating the generation of reports and dashboards to ensure timely and consistent delivery of information.
Interactive Reports:
Building interactive reports that allow users to explore and interact with data to derive insights.
Data Storytelling:
Presenting data in a narrative form that tells a compelling story, making it more understandable and relatable to a broader audience.
Customization:
Tailoring reports to the specific needs and preferences of different user groups or stakeholders.
Distribution and Sharing:
Establishing mechanisms for sharing reports and insights with relevant individuals or teams.
Alerts and Notifications:
Setting up automated alerts and notifications based on predefined thresholds or conditions.
Version Control:
Implementing version control mechanisms for reports to track changes and maintain an audit trail.
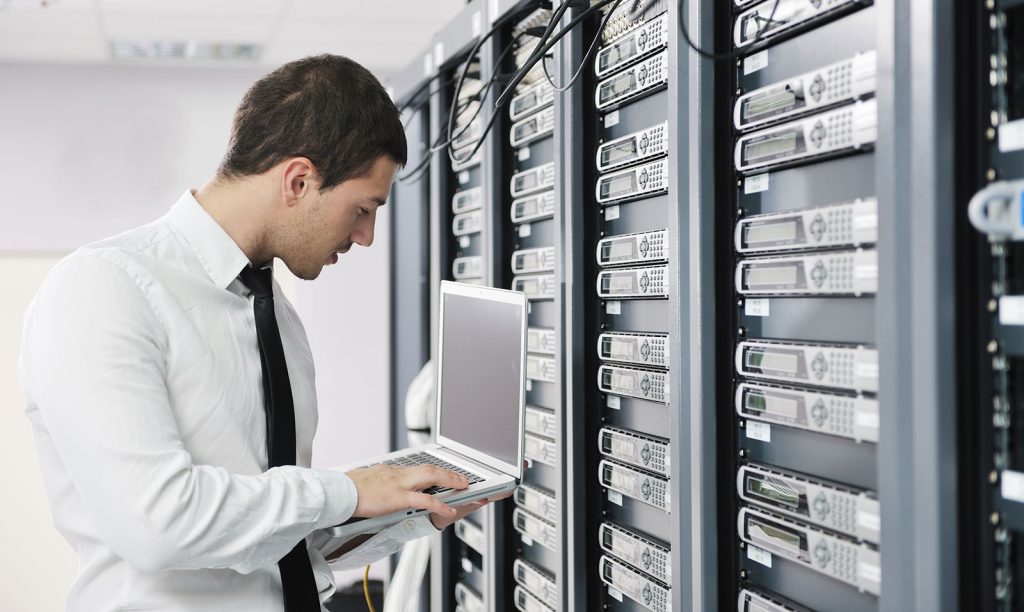
Tools for Data Analysis and Reporting:
Data Analysis Tools:
- Statistical Software: R, Python (with libraries like pandas, NumPy, and SciPy)
- Business Intelligence (BI) Tools: Tableau, Power BI, QlikView
- Data Science Platforms: Jupyter Notebooks, Google Colab
Data Visualization Tools:
- Charting Libraries: Matplotlib, Seaborn, D3.js
- Dashboard Tools: Tableau, Power BI, Google Data Studio
Report Generation Tools:
- Microsoft Excel: For basic reporting and analysis.
- Reporting Platforms: Crystal Reports, SSRS (SQL Server Reporting Services)
Business Intelligence Platforms:
- Tableau: Allows for interactive data visualization and exploration.
- Power BI: Microsoft’s BI tool with robust reporting and dashboard capabilities.
Collaboration and Communication Tools:
Microsoft Teams, Google Suite, Slack, or Email: Communication and collaboration platforms for sharing insights and discussing findings.
Ready to get started?
Best Practices:
Define Objectives:
Clearly define the objectives and goals of the analysis to guide the process.
Understand the Audience:
Tailor reports to the needs and comprehension levels of the intended audience.
Data Quality Assurance:
Ensure data quality through thorough cleaning and validation processes.
Iterative Analysis:
Conduct iterative analysis, refining approaches based on initial findings and feedback.
Documentation:
Document the analysis process, methodologies, and assumptions for reproducibility and transparency.
Data Governance:
Implement data governance practices to ensure data accuracy, security, and compliance.
Usability and Accessibility:
Design reports and dashboards with a focus on usability and accessibility for diverse user groups.
Training and Support:
Provide training and support for users to effectively interpret and use reports.
Security Measures:
Implement security measures to protect sensitive data and restrict access to authorized individuals.
Effective data analysis and reporting contribute to informed decision-making, improved business performance, and a deeper understanding of organizational trends and opportunities. By following best practices and leveraging appropriate tools, organizations can unlock valuable insights from their data to drive success and innovation.
Need More Information?
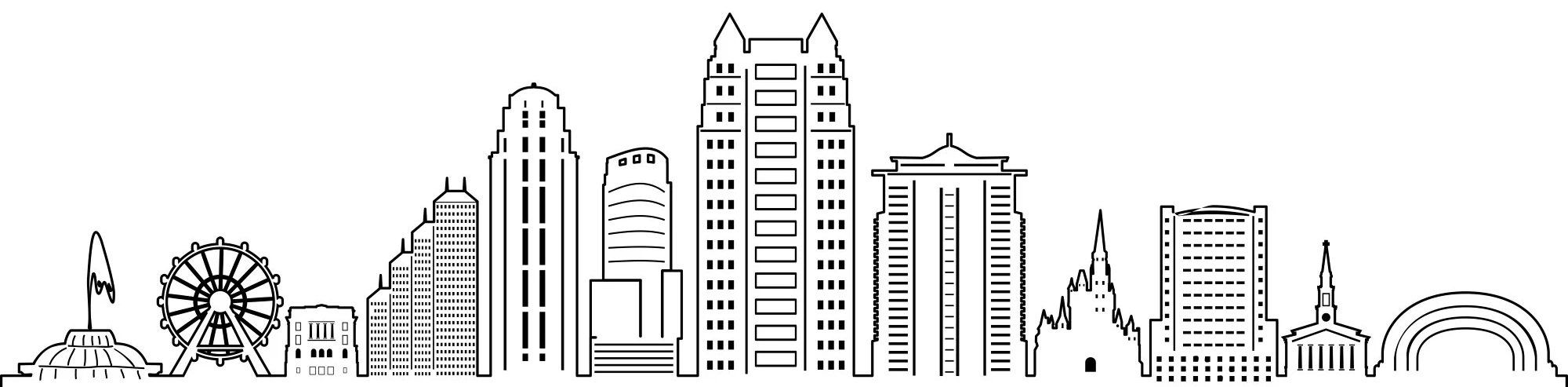